Augmented Work: The AI Teammates Are Coming
How AI and humans learn to work together over time
The collaboration between humans and AI is no longer science fiction and will become an everyday reality sooner than later. From healthcare to creative design, AI systems are increasingly working alongside humans as teammates rather than mere tools. But how do these partnerships develop over time? How do they adapt to challenges? And what makes some human-AI teams thrive while others struggle?
Earlier today, I stumbled upon a new paper by Wang and colleagues that’s trying to find answers to a lot of these questions. Through the lens of "human-agent teaming" (HAT), they are offering their perspective on how these collaborations might evolve. But rather than viewing HAT as a static arrangement, they approach it as a dynamic process that unfolds over time ("process dynamics perspective”). Let’s take a closer look ourselves.
Beyond the Tool Paradigm: AI as Teammate
For decades, our relationship with technology has largely followed a tool-user paradigm - humans wielded technology like a hammer or operated it like a vehicle. But today's AI systems, particularly those powered by large language models, possess unprecedented levels of autonomy, social capability, and proactiveness.
This has sparked interest in a new paradigm: human-agent teaming. In HAT, AI systems aren't just passive tools but active participants that share goals, distribute responsibilities, and engage in ongoing coordination with their human counterparts.
As the authors note: "Human-agent teaming (HAT) is defined as a collaborative framework in which humans and agents pursue shared goals, distribute responsibilities, and engage in ongoing coordination and negotiation to achieve joint objectives."
If one agrees with this perspective or not, current AI definitely feels different and this will ultimately change how we design and study human-AI interaction. Rather than focusing solely on usability or performance, researchers now need to consider team dynamics, shared understanding, and adaptive capacity.
From a practical point of view, we’ve only just begun to make the shift from static AI workflows to AI agents to Multi-Agent Systems. It’s already clear that AI (agent) orchestration will become a very important topic in the near future - but we often ignore the human factor when talking about these systems. I would argue, however, that humans will remain to play a key role in a lot of real-world settings, especially in high risk domains. So thinking about Multi-Agent Systems in isolation won’t be enough - and frameworks like HAT try to fill this gap.
The T4 Framework: A Lifecycle View of Human-AI Teams
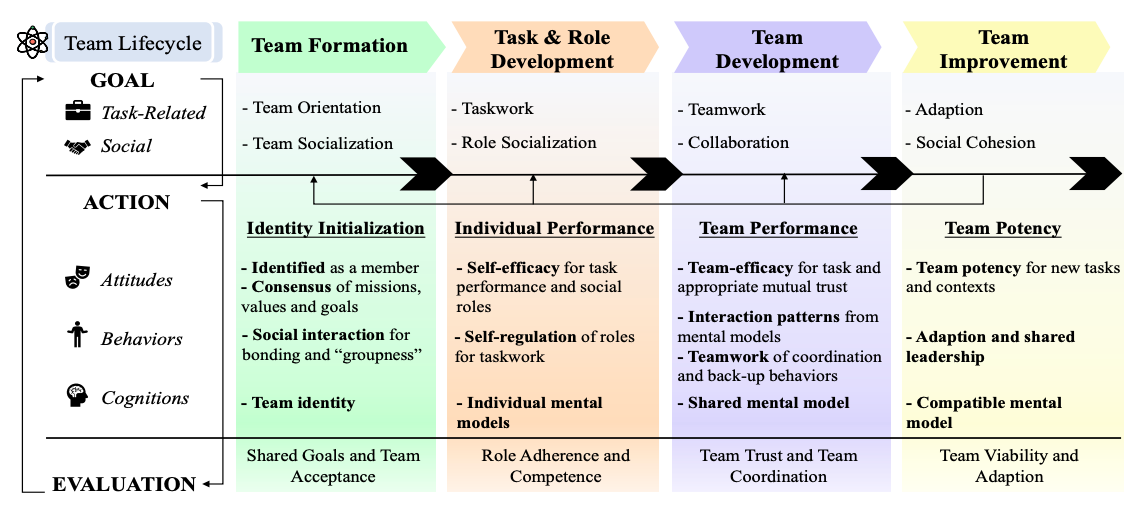
One of the researchers’ core contributions is the T4 framework – a comprehensive model that views HAT through four developmental phases:
Team Formation: Establishing team identity and shared goals
Task and Role Development: Defining who does what and building individual competence
Team Development: Creating shared understanding and effective coordination
Team Improvement: Building adaptability and long-term viability
What makes this framework powerful is how it integrates two key dynamics:
Task dynamics: The cyclical process through which team members set goals, execute tasks, evaluate outcomes, and adjust strategies
Team developmental dynamics: The iterative progression through the four phases
These dynamics don't operate in isolation but continuously influence each other. As the team completes tasks, it progresses through developmental phases. Meanwhile, each phase shapes how the team approaches its tasks.
The ultimate goal? A self-managing, self-regulating team capable of adapting to new challenges without external intervention.
Keep reading with a 7-day free trial
Subscribe to LLM Watch to keep reading this post and get 7 days of free access to the full post archives.